Niresh Rajah, Chief Data & AI Officer at DLA Piper - The Future of Data & AI
- James Pepper
- Feb 7
- 9 min read
Updated: 5 days ago
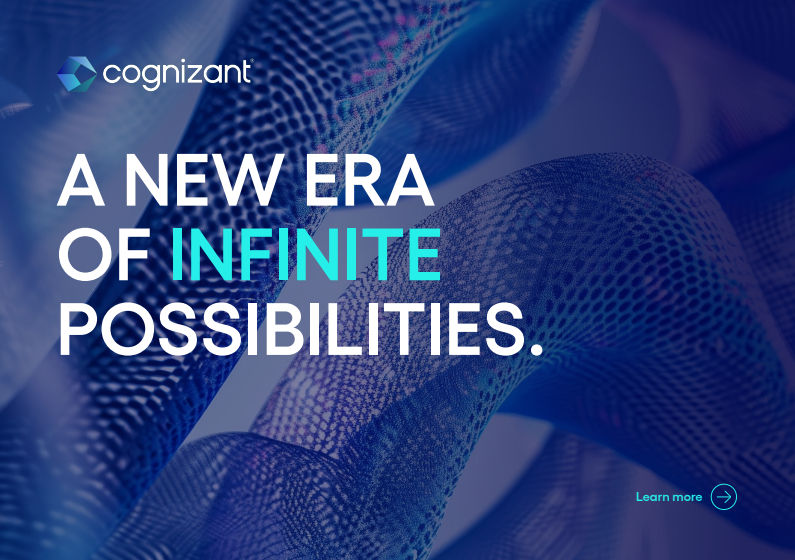
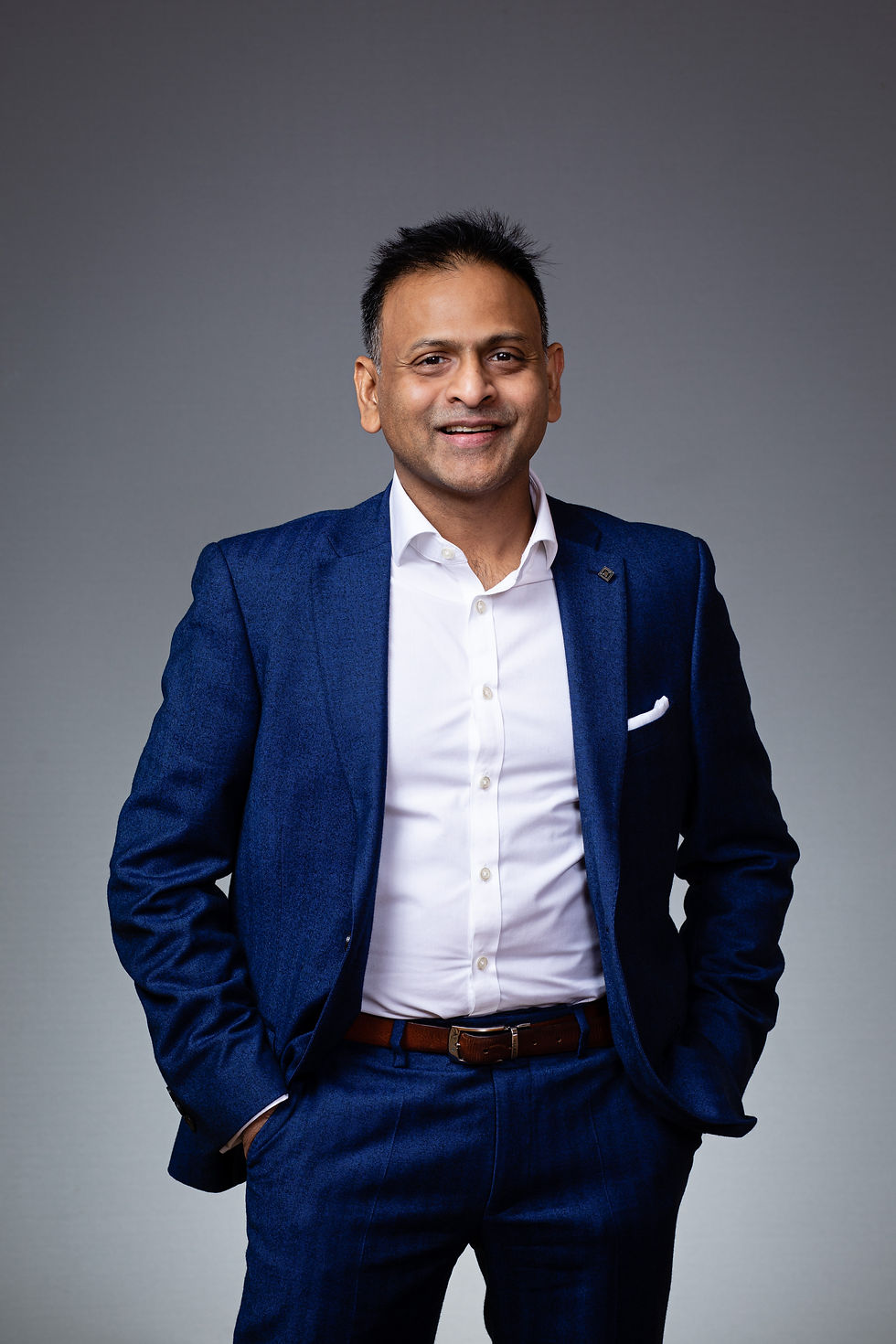
JP: I met with Niresh for coffee in a cosy lounge at the Sun Street Hotel in Shoreditch, for a discussion around the future of data and AI in financial services, and the impact of Gen AI.
Here's what he had to say...
JP: Defining Impact: How do you see Gen AI reshaping how organisations harness and analyse data for strategic decision-making?
NR: As you know, Gen AI relies heavily on vast amounts of ‘available’ , accessible and contextualised high-quality data. I don’t subscribe to ‘Data as the new Oil’ sentiment especially as we strive to move to a sustainable source of energy, however Data is the ‘lifeblood’ which fuels these AI systems. The availability (when correctly contextualised and accessible) of extensive and diverse datasets allows large language models (LLM’s) to learn patterns, generate insights, and provide innovative solutions especially in ‘knowledge rich’ environments .
I firmly believe in the symbiotic relationship between Data & AI – the strength of the data foundation results in the outcomes and benefits to be realised by AI/Gen AI.
I have seen throughout out my career the focus on being data driven and strategic decision making being attuned to decisions driven by data. Whereas we have been using analytics and visualisation to tell the story backed up by Data, the nuance of AI undertaking sophisticated data interpretation and predictive analytics enables trends and correlations to be formulated on markets, revenue/profitability , clients etc where the unsupervised learning of these models do result in some insightful interpretations which isn’t immediately apparent with traditional data analytics.
JP: Real-World Applications: Can you share examples of how generative AI is leveraged innovatively ?
NR: I can talk about the two industries which I have been working in extensively since Gen AI exploded into the scene in Q1 2023 – Financial Services and Legal Services, both regulated, knowledge intensive industries. The knowledge rich industries are prime for automation (which has been going for a number of years especially in Financial Services), and more recently Gen AI.
In Financial Services, it is the development of detailed financial and risk models, performing risk assessments, and generating investment strategies based on historical and real-time data. This is especially pertinent where credit risk and model risk calculations for lending, liquidity and basis of capital ratios are based on a number of permutations and correlations dependent on modelling multiple data sets. More broader, there has been more doubling down on the use of LLM’s to review customer journey interactions, sentiment, complaints and customer interactions to accurately predict the scenarios from more advanced chatbots to the decision trees to enable a call-back and customer reach out.
The Legal industry has not been traditionally a bastion of innovation and utilisation of automation etc to simplify the workflow. The post Gen AI world resulted in a disruptor of immense proportions for the industry which has been clients of law firms increasingly asking questions on the use of Technology and innovation.
There are multiple use cases including the the basis of contextualised knowledge rich legal research, the preparation for and analysis in due diligence and fundamentally in the drafting, review of legal documents especially complex contracts. In the knowledge and document heavy industry such as Legal , there is also a key area of efficiency and productivity where huge voluminous documents can be summarised together with a chatbot functionality to interrogate documents to pull out specific answers to questions and descriptions of clauses etc through the advent of ‘Legal AI Assistants’.
Given time actually is money in the Legal world , increasing efficiency and reducing the time required for basic and complex tasks is readily becoming the differentiator.
JP: Data Foundation & AI: We hear that Data Foundation is essential for Generative AI play, can you bring that to life for us?
NR: I do think there is a huge misunderstanding at all levels of organisations that somehow AI is the ‘magical bullet’ and just by purchasing the latest AI Software licences (at significant licence costs mind you), that the key use cases, challenges or innovation would immediately appear as if by magic.
This obviously comes from a number of a sources; one the technology vendors themselves extolling a fantastic UX/UI (front end) and user experience and the best presentation the uninitiated have ever seen. The second is that Board members suffer from ‘Board FOMO’, where the innovation by press release has convinced them that their firm needs to be on the bandwagon as fast as possible. Last and not least of course are the multiple consulting firms whipping up myths around benefits and time to market to launch scaled AI programmes through AI products!
In reality, establishing and leveraging the Data Foundation is a critical enabler for AI and Gen AI use cases. Data Foundation in simplest terms is the coupling of good old fashioned Data Management with an enterprise level cloud Data Platform. Data Management of course, covers all aspects of how to organise, curate and align the most critical data an organisation has covering client, employee, financial, product, and transactional data sets and where applicable relevant third party data. The focus is to understand and catalogue the most important data and organisation has and then ensure the quality of the data, and establish processes so the providence of that data can be mapped and leveraged in a repeatable automated manner.
The second part of the couple , data platform does require the purchasing and provisioning of one of the leading cloud based data lake/ data warehouse technologies to ingest, transform, store and orchestrate the data in a manner by which it can be consumed for analytical or data science purposes to create value. Leading Data Platforms enable inter-operability with latest data analytics, data catalogue, ML Ops applications and data visualisation tools, etc.
It goes without saying for any Gen AI use cases, the foundational models rely upon sound Data Foundation to deliver the outcomes and benefits…..this is being increasingly called ‘AI ready Data’. By maintaining high data quality, organisations can trust the insights generated by AI, leading to more reliable and actionable outcomes.
JP: Ethical Considerations:Ethical Considerations: With the rapid adoption of generative AI, how do you address concerns about ethical data use and algorithmic bias?
NR: This is the most critical aspect of AI and Gen AI – how do we address the ethical data use, algorithmic bias and Intellectual Property rights especially in creative industries. Building on the previous items we discussed on Data Foundation, the data sets used for training the AI models are fundamental in the potential biases created in the algorithm and added to this is selection of the appropriate foundational models in Gen AI. The foundational models (very well known examples such as Open AI’s GPT-3 and GPT-4 for Chat GPT) which can be built on top of for a variety of use cases and applications and hence selection and curation are important.
Addressing these concerns requires a multi-faceted approach that encompasses robust data governance, transparency, and diversity. A comprehensive data governance framework is essential for ensuring that data is used legally and ethically . Aligned to the Data Foundation discussed we have just had, this involves creating policies and controls that govern data collection, storage, access, and usage. Establishing clear rules and guidelines on data privacy, consent, and security to protect individuals’ rights and maintain trust is fundamental.
Transparency is vital for building trust in AI and Gen AI models, and hence documenting and being transparent of how AI models are developed, trained, and deployed. This includes providing insights into the data sources used, the methodologies applied, and the decision-making processes of the AI/ Gen AI applications and use cases. This is one of the reasons I am sceptical of black box Gen AI solutions bought off the shelf and not one which we as the host organisation can have full explainability of the AI models.
As we have already seen some of the issues with Gemini and other AI Models, ongoing monitoring, sample testing and human in the loop to continuously review any bias in the algorithms is important.
JP: Human-AI Collaboration: How can organisations balance relying on AI-driven insights and maintaining human oversight in data-driven decisions?
NR: This is interesting and we can already see the divergence which the U.S is taking with regard to the European Union in terms of regulation. Its important to incorporate human-in-the-loop mechanisms where people can validate and refine AI-driven insights and outcomes. The design of the AI/Gen AI systems and how the models are trained, grounded in which data sets and the contextual understanding are the aspects ‘Humans’ fundamentally need to play a role. For some use cases, AI can be tasked with data processing, pattern recognition, and generating insights, while humans should focus on interpreting these insights, considering context, and making final decisions. It is the interpretation and decision making which are the crucial aspects. This leads onto AI and Data Literacy for those with roles to be the human interface in this process. Good to encourage data literacy across all level of the organisational and train/coach employees with the skills to understand and interpret AI-generated insights.
Balancing AI-driven insights with human oversight is crucial for making informed and ethical decisions. By establishing clear roles, ensuring transparency, promoting data literacy, and fostering a collaborative culture, organisations can harness the power of AI while maintaining the critical element of human judgement.
JP: Data-Driven Culture: What are the key steps to fostering a data-driven culture that embraces change and innovation at all organisational levels?
NR: This is something that all CEO’s and Boards are referencing ‘being Data Driven’ without necessarily understanding the investment, patience, commitment and changes in responsibilities and cultural change necessary to achieve sustainable success. As you become more senior at ExCo’s and Board’s, it is also something which is more and more difficult to do as ‘experience’, ‘gut instinct’ and good old fashioned hubris gets in the way.
So how does one establish a data driven culture; first by setting a clear vision and direction that we are going to be enable data driven decision making and, second by walking the walk or eating one’s own dog food as a previous leader used to tell me, which involves senior leadership meetings where decisions are continuously made utilising data as an input. By the senior leadership team making decisions using data and also the stories which emerge about how decisions are being made utilising this data at the top of the house – sends a very clear message to everyone in the company.
Thirdly, being data driven from putting together numerical values and developing data visualisation using some of the readily available tools such as Power BI and then the story-telling which comes with reading and interpreting the data is both an art and science simply termed ‘Data literacy’ and this needs to be trained (formal training ) and coached. We all get better at this by doing this more and more and making it an inherent part of our meetings and papers.
The challenge is then to drive adoption whereby through ongoing data transformation techniques – the adoption of data driven business cases, ongoing validation, role model behaviour from senior folk, and publicly rewarding data driven decision making starts to make an impact. The impact of data driven approach needs to be measured, tracked and those powerful stories continue to be told. Finally and importantly , data driven cultural change is a long game and we need the Board and ExCo to be patient to see the benefits come through.
JP: Overcoming Resistance: How do you address resistance to AI and data-driven change, particularly from teams or individuals accustomed to traditional methods?
NR: Everyone is coming to grips with Generative AI and the impact its having on day to day business. The Gen AI foundational models themselves and the applications from the technology vendors and hyperscalers are also moving at break-neck speed. I feel that the biggest challenge isn’t overcoming resistance, its actually filtering through all the noise which is out there to come up with a handful of Gen AI use cases which can deliver actual benefits and then establishing focus to land the pilots and then scale appropriately. Coupled with this is the time that needs to be spent on educating or coaching the impacted individuals on how to leverage the Gen AI use cases appropriately.
Finally it is the continuous story telling and measuring and tracking actual benefits – the delivery of time saved on a task, as an example utilising an AI assistant based on Open AI GPT 4 which we have built, it reduced the time taken on a task from 3 hours to 10 minutes. Delivering on the value and then measuring this in a focused fashion on how it actually helps someone do their job, can do wonders in changing ‘naysayers’ to ‘cheerleaders’.
JP: Future Outlook for AI and Data:What trends or technologies do you believe will define the next wave of AI and data's influence in organisational transformation, and how can companies prepare for them?
NR: I can probably be a very rich man if I was able to predict that ! We are only at the start of the Gen AI revolution, and I think we will see the adoption of the use cases with the firms which are truly committed in this journey both in commitment and investments, actually translating the use cases for efficiency, revenue generations, customer acquisition at much greater scale in 2025/2026 with tangible benefits. The pace of technological innovation and change with the hyperscalers is so vast that I am expecting Agentic AI to make the giant leap forward to revolutionise work-flow in business. Agentic AI whereby the AI takes autonomous decisions and selects the best response and best ‘AI Models & Engines’ to use for each problem or challenge adapting in real-time with an understanding of context and objectives will be revolutionary and what we will then experience the real intelligence in artificial intelligence.
I see the practice of Data through ‘enhanced Data Foundations’ really taking to the forefront so that AI ready Data is developed and optimised. Similar to BCBS 239 in the Financial services sector which really brought the professionalism of Data Management to the forefront, I think the advent of Data Foundation for Gen AI purposes will result in huge investments and focus on Data Platforms, Data Management & Governance, Architecture and Data Engineering.
As we have discussed, data is indispensable for generative AI, especially in knowledge-rich industries. By effectively harnessing and managing data, organisations can unlock the full potential of AI to drive innovation, efficiency, and strategic advantage.
Comments